Exploring the Next Frontier: LLMs and AlphaFold's Impact on Medicine
Written on
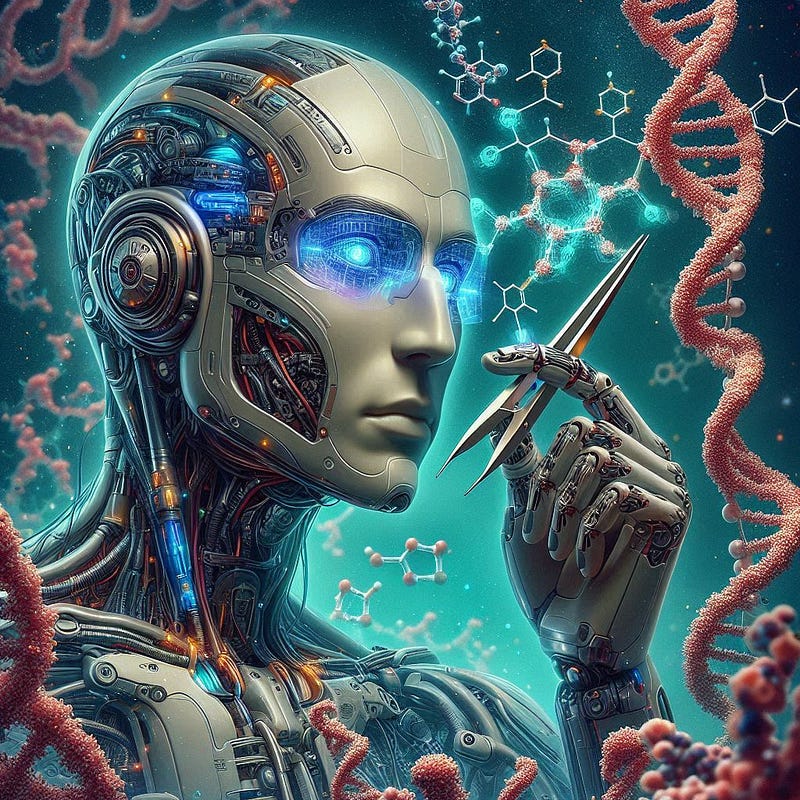
In the words of Linus Torvalds, “In real open source, you have the right to control your own destiny.” With the arrival of AlphaFold3, the response was not as enthusiastic as anticipated by DeepMind. Nevertheless, this is yet another chapter in the ongoing transformation within biological sciences. Large Language Models (LLMs) are significantly altering both the medical field and pharmaceuticals. Three years after AlphaFold2's debut, computational biology has undergone substantial changes that warrant reflection.
- How does AlphaFold3 signify a pivotal moment, both positively and negatively?
- What led to the disappointment among researchers?
- What shifts are occurring within the community’s response?
- What prospects do LLMs hold for the future of biology?
This article delves into these questions.
The Launch of AlphaFold3
When AlphaFold2 was introduced, it seemed to herald a new era. For almost a century, accurately predicting a protein's structure based solely on its sequence was perceived as unattainable. For nearly fifty years, computational biology grappled with understanding the intricate rules governing protein formation.
In 2021, AlphaFold2 appeared to have cracked the code. The model showcased unprecedented accuracy in its predictions, igniting excitement within the scientific community regarding its potential applications in both research and industry.
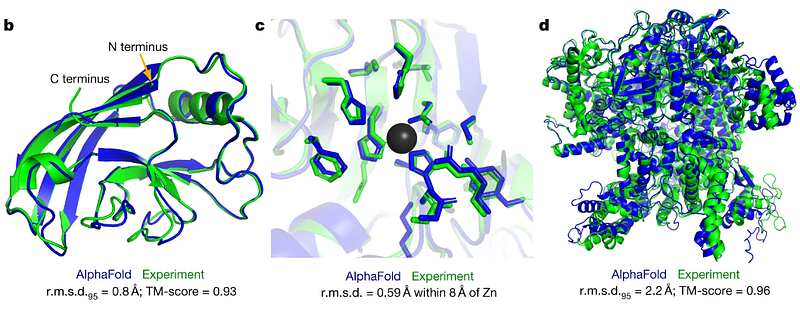
Recently, the scientific realm buzzed once more as DeepMind unveiled AlphaFold3. One might wonder why a new version was necessary if the protein-folding conundrum had already been resolved.
In truth, predicting protein folding was merely the start of a broader journey. AlphaFold2 achieved remarkable precision in modeling single-chain proteins. However, this only scratched the surface of the protein folding challenge and the scientific pursuit of understanding protein structures.
Since AlphaFold2’s launch, various teams have sought to expand its capabilities. A notable advancement, AlphaFold-Multimer, was developed to assess protein complexes.
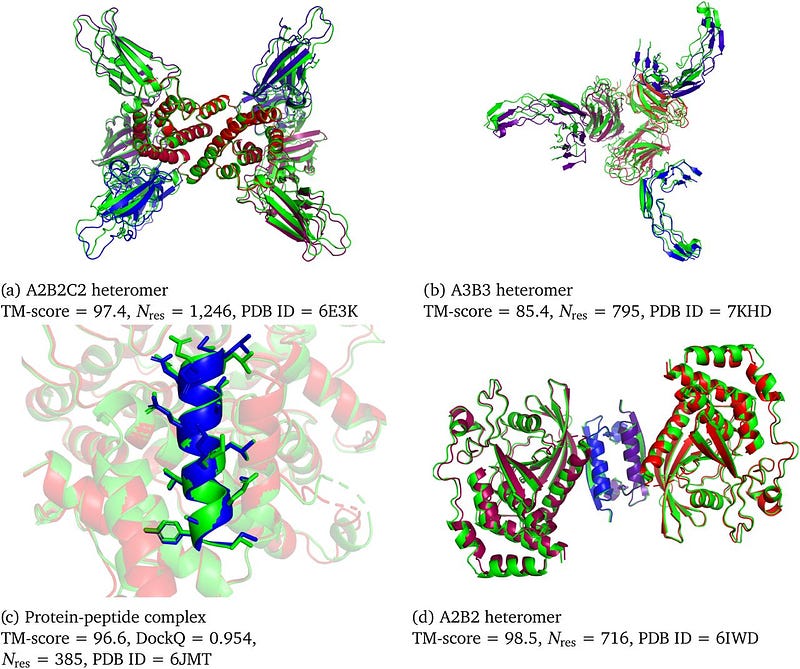
This success raises the question: can we accurately forecast the structures of complexes that involve a diverse range of biomolecules, such as ligands, ions, and nucleic acids, using a deep learning framework?
One limitation of AlphaFold2 was its inability to forecast interactions. This shortcoming is critical, as proteins are central to an intricate ecosystem characterized by continuous interactions among themselves and with various macromolecules. Furthermore, comprehending how drugs interact with biological molecules is essential in drug development. Designing drugs that bind effectively to target proteins is a primary aim in computational chemistry, making it logical that AlphaFold3 was developed to address this need.
Despite its technical sophistication, AlphaFold3 is a noteworthy achievement. It minimizes reliance on multi-sequence alignment—previously necessary for predicting protein interactions—and introduces a novel Diffusion Module for structure prediction. In essence, AlphaFold3 streamlines the process while enhancing overall performance. However, leveraging a generative diffusion approach presents its own challenges, including the risk of generating inaccurate results.
AlphaFold3 combines features from models designed to predict specific interactions, effectively serving as a generalized model capable of predicting various types of interactions. Moreover, its resolution is significantly improved.
In summary, AlphaFold3 demonstrates superior performance compared to many existing software tools in predicting protein structures and their interactions.
However, a lingering question remains: Will AlphaFold3 achieve the same groundbreaking impact as its predecessor?
The Community's Discontent
“We must find a balance between ensuring accessibility and making an impact on the scientific community without undermining Isomorphic's commercial drug discovery efforts.” — Pushmeet Kohli, DeepMind’s head of AI science.
Access to AlphaFold3 is restricted to a dedicated server. Users can submit their protein sequences and receive predictions within ten minutes, but are limited to ten predictions per day. Additionally, structures of proteins bound to potential drugs are not available, and usage is confined to non-commercial purposes.
In stark contrast, AlphaFold2's entire code was made publicly available upon its release. AlphaFold3, however, comes with only 'pseudocode', which, regardless of its detail, poses challenges for replicating the model.
Prestigious research journals typically require authors to share their code or at least commit to providing it upon request. In this instance, it was evident from the outset that the code would not be available.
This decision has incited backlash from the scientific community, culminating in a public letter of condemnation:
“We were disheartened by the absence of code, or even executables, accompanying the publication of AlphaFold3 in Nature. While AlphaFold3 expands on AlphaFold2 by incorporating small molecules, nucleic acids, and chemical modifications, it was released without the means to validate or utilize the software in a high-throughput manner.”
In response to the backlash, Nature published an editorial explaining its reasoning for allowing AlphaFold3 to be published without code or model availability.
Despite justifications from both Google and Nature, the scientific community remains dissatisfied. The restrictions imposed by Google and the lack of model release significantly hinder its potential impact and usefulness. The inability to assess the model's quality limits its application for new research avenues, unlike its predecessor.
Perhaps the criticism has influenced DeepMind to commit to releasing the model for academic purposes, but the damage may already be done.
Consequently, the community feels betrayed. This same community that once celebrated AlphaFold2's release now finds itself disappointed by its successor.
How will this community react? What does the future hold?
The Prospects for LLMs in Biology
AlphaFold2 ignited a revolution, but it isn't the only significant player. Following its release, other models capable of accurately predicting protein structures emerged, capturing the attention of major companies like META with ESMfold and Salesforce’s ProGen. These models were released as open-source and quickly embraced by the scientific community.
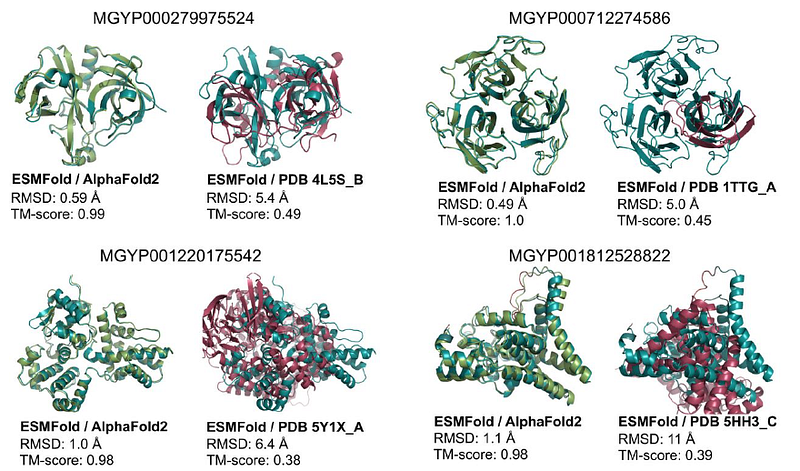
Despite being slightly less accurate than AlphaFold2, their impact has been remarkable. They are more user-friendly (in part due to their transformer-based designs) and are readily available on HuggingFace. Additionally, ESMfold offers a significantly faster and more cost-effective inference process compared to AlphaFold2.
AlphaFold2 is undeniably a technical wonder, but it presents challenges for applications beyond protein structure prediction. Fine-tuning it demands considerable resources and expertise. Conversely, the newer models are more lightweight and easier to fine-tune.
Researchers have recognized this distinction, particularly when developing additional applications that require model modifications or fine-tuning. In biomedical science, genuine success hinges on the community's adoption of innovations: models must be replicated, utilized, adjusted, and tailored.
This eagerness among researchers indicates a strong interest in utilizing these models to create innovative applications. If a model fails to meet expectations or proves cumbersome, it risks being abandoned, leaving behind a vast graveyard of unmaintained bioinformatics libraries.
Yet, why have only models released by major corporations been open-source thus far?
The expense of training these models is substantial. Training a model like AlphaFold3 can cost up to a million dollars in cloud computing resources. Having the code is merely the first step; one must also download and process intricate datasets and navigate the technical challenges of model training.
Nevertheless, the costs associated with these models and their training are ultimately manageable. Some institutions can cover the expenses, and the open-source community has successfully tackled even more complex projects in the past. However, two factors must be considered. First, AlphaFold2 left the community in awe; few anticipated that a computational model could achieve such results in protein folding. Second, protein folding and computational chemistry have historically been closely tied to experimental environments.
Today, however, academic research is better equipped, with many researchers possessing the computational skills necessary to train open-source models. The quest to recreate AlphaFold3 in an open-source format has already begun. The OpenFold consortium is actively working on developing an OpenFold3 model, and the University of Washington is pursuing a similar objective, along with independent initiatives like lucidrains.
Beyond merely recreating AlphaFold3, several open-source models tailored for health-related applications have emerged recently. Researchers are fine-tuning these models to generate new sequences, leading to innovative solutions such as Protein-Language models that integrate LLMs with protein modeling.
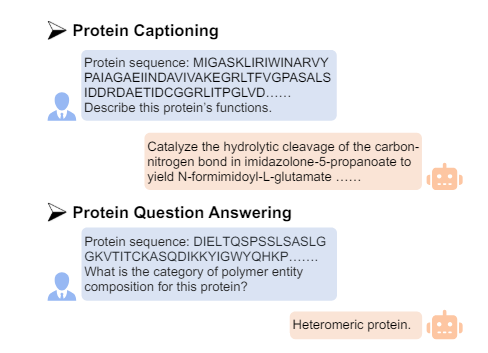
Another noteworthy application of LLMs in biology is ScGPT, which focuses on generating representations of single-cell data, aiding in target discovery within the drug design process.

Numerous other models have been developed for specialized biological and medical tasks, encompassing histology, gene regulation, expression, pathways, and beyond. Researchers are even experimenting with various LLM types, including state-space and RNN-based architectures.
These models indicate emerging trends for the future. Open-source models are increasingly being utilized for medical and biological applications. Initially, models like AlphaFold2 and ESMfold were primarily used for inference to predict protein structures, which biologists then employed in their publications or for hypothesis generation. In the subsequent phase, researchers began to fine-tune these models for specific tasks, combining them as needed to meet different requirements.
We are now entering a new stage. Models like scGPT demonstrate that the community is moving beyond simple inference or modification; they are developing entirely new models when existing ones fall short.
These developments lay the groundwork for a revolution. Researchers no longer need to rely solely on large corporations to create new models; they possess the skills and resources to develop them independently. This capability is particularly beneficial in the biomedical realm. As a result, we anticipate a surge of specialized models tailored for research applications, many of which will be built from the ground up. While major companies will continue to focus on foundational models, researchers now have the expertise to develop models for applications that may not align with the interests of larger firms.
To summarize, companies like Google and META will persist in training models for structural predictions (we may soon see an AlphaFold4). Well-funded groups will strive to create open-source alternatives (such as RoseTTAFold). Increasingly, researchers and biotech firms will adapt published models or create new ones tailored to their needs.
This indicates that AlphaFold2 was the catalyst that ignited the scientific community, which is now primed to advance the revolution.
Final Thoughts
AlphaFold3 serves as a “cautionary tale” for academics regarding the risks of relying on technology firms like DeepMind for developing and distributing crucial tools. “While it’s commendable that they accomplished this, we must not become dependent on it,” remarks AlQuraishi. “We need to establish a public-sector infrastructure to facilitate this within academia.”
Predicting protein structures has vast implications for biomedical applications. It can be utilized for a myriad of purposes, including understanding pathogen-host interactions, developing innovative drugs, creating new vaccines, and exploring molecular changes linked to cancer. This revolution also extends to areas such as designing enzymes to mitigate environmental pollution and engineering crops to withstand climate change. If this revolution remains monopolized by a select few companies, we will only witness a fraction of its potential benefits.
AlphaFold2 has validated the feasibility of predicting a structure from its sequence. However, it is not the endpoint of research, as it still has multiple limitations. AlphaFold3 addresses some of these limitations, but its inaccessibility curtails its usefulness.
This revolution can be delineated into three phases:
- In the first phase, researchers utilized LLMs as they were, primarily relying on inference through dedicated servers, mainly to generate scientific hypotheses.
- The second phase involved modifying or adapting the models for specific cases. Researchers fine-tuned models with proprietary data or extracted representations for various applications. As more models became available, the community began to combine them into increasingly sophisticated pipelines.
- In the third phase, numerous teams started creating models from scratch, recognizing that training a new model could be more cost-effective than modifying an existing one.
Each of these phases reflects an increase in both expertise and resources devoted to employing LLMs in biology. Initially, the community may have been taken aback by AlphaFold2’s impact, but it swiftly gained expertise and began investing in LLMs. As a result, many researchers today are creating customized models for their specific needs.
This newfound expertise enables researchers to develop LLMs tailored to their specialization. For instance, those focusing on monoclonal antibodies can create LLMs designed to generate sequences of new antibodies. They may choose to fine-tune an existing model, combine it with a language model for conditional generation, or develop a new one from the ground up. Once they have their model, they can quickly test results in the lab and refine training as necessary. This model diversification will yield experimental benefits, with new data generation used to train future models.
Undoubtedly, major companies like Google and META are investing in biology LLMs. However, a burgeoning ecosystem of biotech firms and academic labs can help realize the full potential of LLM applications in biology, which necessitates open-source models for unrestricted community use. Today, the scientific community is much better equipped for this challenge, and it is likely that open-source models will dominate the landscape moving forward. This will facilitate the development of diverse models in both structure and function, given that expertise is now more widespread.
TL;DR
- AlphaFold2 represented a pivotal moment in demonstrating the impact of LLMs in biology and medicine.
- Its open-source release significantly contributed to its influence.
- AlphaFold3 is unlikely to have the same effect due to its closed-source nature, limiting researchers' ability to utilize it freely.
- Open-source models empower the community to innovate, combine, and generate new hypotheses.
- The research community, once passive in its approach, is now actively engaging with published models, underscoring a robust open-source movement.
- In the future, only open-source LLMs will gain genuine traction among researchers, leading to their modification and adaptation for countless applications, while proprietary models may be disregarded.
What are your thoughts? How do you foresee LLMs contributing to biology? Share your insights in the comments!
If you found this information valuable:
Connect with me on **LinkedIn* and explore my other articles. Check out this repository for weekly updates on ML & AI news. I welcome collaborations and projects, and you can reach me on LinkedIn. Subscribe for free to be notified when I publish new articles.*
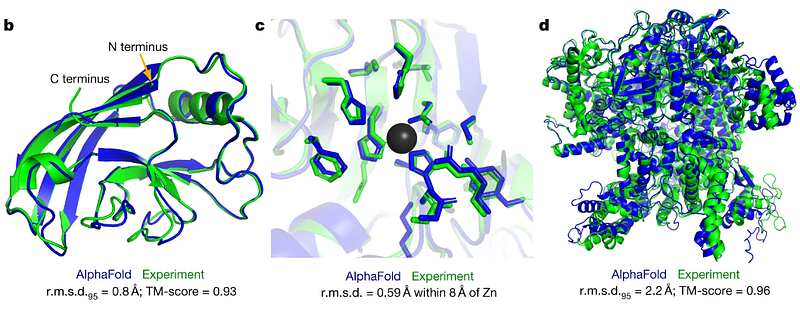
References
Here is a list of key references consulted in writing this article:
- Jumper, 2021, Highly accurate protein structure prediction with AlphaFold, link
- Abramson, 2024, Accurate structure prediction of biomolecular interactions with AlphaFold 3, link
- AlQuraishi, 2021, Protein-structure prediction revolutionized, link
- Evans, 2021, Protein complex prediction with AlphaFold-Multimer, link
- Lin, 2022, Language models of protein sequences at the scale of evolution enable accurate structure prediction, link
- Nature, 2024, AlphaFold3 — why did Nature publish it without its code? link
- Callaway, 2024, Major AlphaFold upgrade offers boost for drug discovery, link
- Yang, 2023, AlphaFold2 and its applications in the fields of biology and medicine, link
- Bertoline, 2023, Before and after AlphaFold2: An overview of protein structure prediction, link
- Xu, 2023, Toward the appropriate interpretation of Alphafold2, link
- Madani, 2023, Large language models generate functional protein sequences across diverse families, link
- Ruffolo, 2024, Design of highly functional genome editors by modeling the universe of CRISPR-Cas sequences, link
- Liu, 2023, ProtT3: Protein-to-Text Generation for Text-based Protein Understanding, link
- Nechaev, 2024, Hibou: A Family of Foundational Vision Transformers for Pathology, link
- Cui, 2023, scGPT: Towards Building a Foundation Model for Single-Cell Multi-omics Using Generative AI, link
- Della Torre, 2023, The Nucleotide Transformer: Building and Evaluating Robust Foundation Models for Human Genomics, link
- Avsec, 2021, Effective gene expression prediction from sequence by integrating long-range interactions, link
- Schiff, 2024, Caduceus: Bi-Directional Equivariant Long-Range DNA Sequence Modeling, link