Exploring the Paradox of Understanding in AI: A Deep Dive
Written on
Chapter 1: The Intriguing Intersection of AI and Comprehension
In the domain of artificial intelligence and data science, we encounter a compelling dilemma that resonates with the intellectual pursuits of thinkers like Douglas Hofstadter and Richard Feynman. This dilemma encompasses the tension between creation and comprehension, encapsulated in Feynman's insightful assertion:
“What I cannot create, I do not understand.”
However, within the landscape of neural networks and large language models (LLMs), we find ourselves grappling with the paradox that we have engineered algorithms whose underlying mechanisms remain elusive even to their creators.
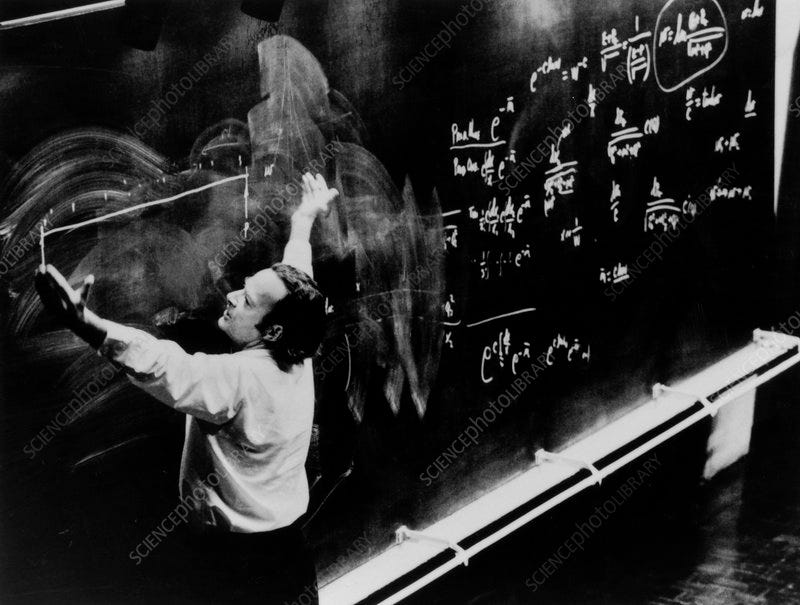
The Mystery of Machine Learning
Machine learning has heralded a new chapter in scientific and technological progress. Its remarkable abilities, from diagnosing illnesses to unraveling intricate environmental trends, seem almost miraculous. Nonetheless, the true essence of these ‘black box’ models, particularly deep learning algorithms, continues to be enveloped in obscurity. These systems analyze enormous datasets, learn patterns, and make decisions, yet the ‘how’ and ‘why’ behind their conclusions often remain hidden, even from the engineers who designed them.
This enigma presents a significant challenge: how can we place our trust in and responsibly utilize technology that we do not fully grasp? This query is not only pertinent to engineers and scientists but also resonates with ethicists, policymakers, and the general populace. The opaque nature of these systems raises pressing concerns regarding accountability, bias, and the transparency of AI-driven decisions that influence human lives.
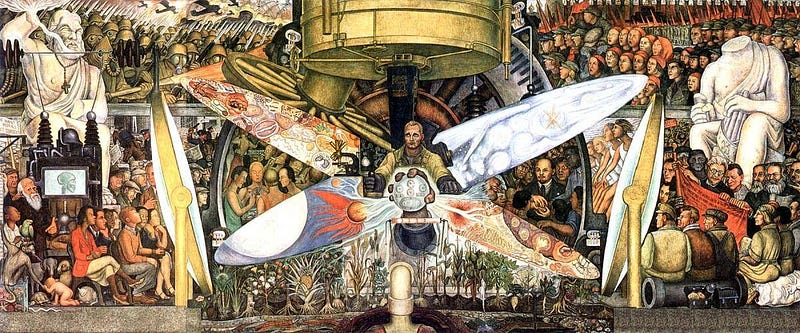
Bridging the Gap Between Creation and Understanding
The quest to decode these sophisticated models is both captivating and essential. It calls for a harmonious blend of technical skill, philosophical inquiry, and ethical contemplation. As we push the boundaries of AI, our responsibility extends beyond mere technological creation to a profound understanding of our innovations. In “Gödel, Escher, Bach: An Eternal Golden Braid,” Hofstadter insightfully notes:
“In a formal system, there can be no such thing as ‘meaning’ in the sense that humans usually use the word. In short, a formal system, no matter how powerful — cannot lead to all truths.”
This observation emphasizes the importance of looking beyond technical expertise. The challenge lies not just in creation but in grasping the broader implications and deeper meanings inherent in our advancements. As we navigate the complexities of artificial intelligence, our journey is influenced by both algorithmic logic and human insight.
It is through this delicate interplay that we begin to understand the essence of our endeavor. Hofstadter’s exploration of cognitive patterns sheds light on this fusion, suggesting that our engagement with AI transcends binary logic and ventures into a domain where understanding is not merely a result but an integral aspect of the creative process.
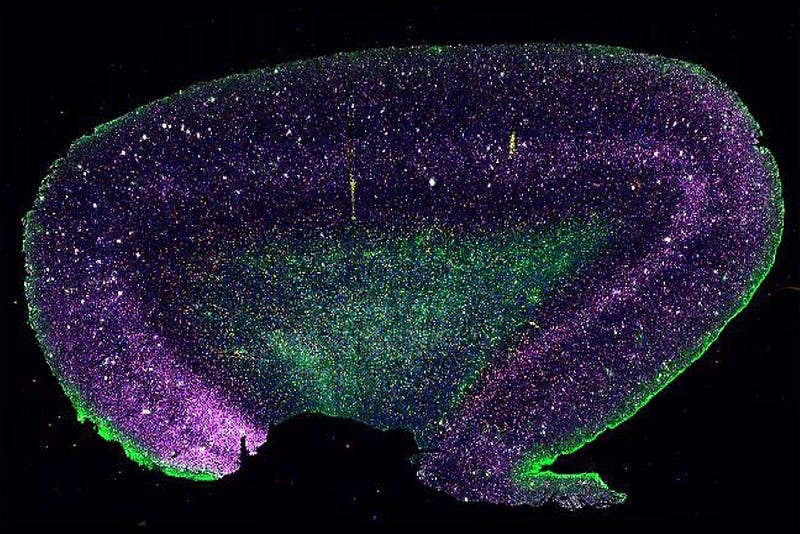
Orchestrating a Transparent Future
As we delve further into the intricacies of AI, our aim should be to convert these ‘black boxes’ into ‘glass boxes’ — systems whose inner workings are not only transparent but easily comprehensible. Such transparency is essential for fostering trust and ensuring the ethical application of AI technologies. This journey from obscurity to clarity is where each technical enhancement is coupled with a deeper moral and philosophical understanding of the technology we harness.
The future of AI is not solely focused on technological advancements; it is about achieving a balance between innovation and insight, between developing sophisticated models and unveiling their mysteries. It involves using AI as a tool for enlightenment rather than a blunt instrument.
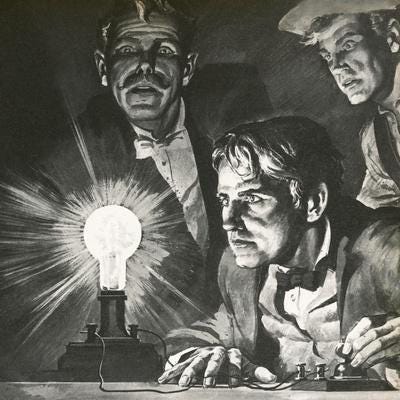
Conclusion: The Symphony of Insight
In the grand symphony of data science and AI, each breakthrough and algorithm contributes to a larger composition. The beauty of this creation lies not only in its harmony but also in our comprehension of its individual elements. As we continue composing this symphony, we must ponder not just how we will shape the future of AI but how we will come to understand it. Steve Jobs once remarked,
“Everything around you that you call life was made up by people that were no smarter than you. And you can change it, you can influence it… Once you learn that, you’ll never be the same again.”
This realization is transformative, urging us to embrace both power and responsibility. It calls us to take an active role in defining the narrative of AI, recognizing that our collective efforts are crucial in guiding this technology toward a future that is not only intelligent but also humane and insightful.
How, then, will we navigate this paradox of creation and understanding to ensure that the AI of tomorrow is not just powerful but also wise and transparent? Or perhaps, how will you?
Thank you for reading!
If you found this article insightful and wish to stay updated on my latest posts, consider subscribing to my email list. If you’d like to express your appreciation, feel free to treat me to a cup of coffee. Your support means a great deal! 😊
Chapter 2: Engaging with AI's Complexity
This first video, titled "Inside the Black Box: Introduction to Explainable AI," delves into the foundational concepts of explainable AI, addressing the complexities that arise in understanding AI systems and their decision-making processes.
The second video, "Interpretable AI: Stop Explaining Black Box Machine Learning Models," features Cynthia Rudin discussing the challenges and potential solutions for interpreting machine learning models, shedding light on the importance of transparency in AI systems.